Research
The medical physics group mainly conducts research on deformable image registration (DIR), CT-based lung function imaging, MRI-Linac, AI-based treatment response/side-effect prediction, AI-based automatic planning, and AI-based patient QA. In particular, we are actively developing radiation therapy planning support systems using advanced medical image processing including artificial intelligence (AI). Technologies such as DIR, AI-based automatic planning, AI-based patient QA, AI-based treatment response/side-effect prediction were alreadly implemented in society through domestic and overseas patents and commercialization. In our laboratory, in order to achieve great research results, the individual research of faculty members and graduate students is well connected like a single line, and all members are conducting research so that we can finally achieve great research results. Research themes for graduate students are also determined accordingly. As a medical physicist, I believe that it is important to feed back the results of my research to the clinic, ultimately leading to improved treatment outcomes and reduced side effects for patients. Below is an overview of our main research projects.
Contents
1. Automation of radiotherapy process using AI: Toward personalized medicine
① Treatment policy decision
② Automatic contouring
③ Automatic treatment planning
④ Automatic patient QA
⑤ Actual delivered dose distribution evaluation
2. Development of new image using AI/DIR
① CT-based lung functional imaging
② Radiation pneumonitis hazard map
3. Clinical evaluation and technical development of automatic contouring and accumulated dose distribution evaluation system using deformable rigid registration
4. Clinical technology research of online adaptive radiotherapy with MR-Linac
1.Automation of radiotherapy process using AI: Toward personalized medicine
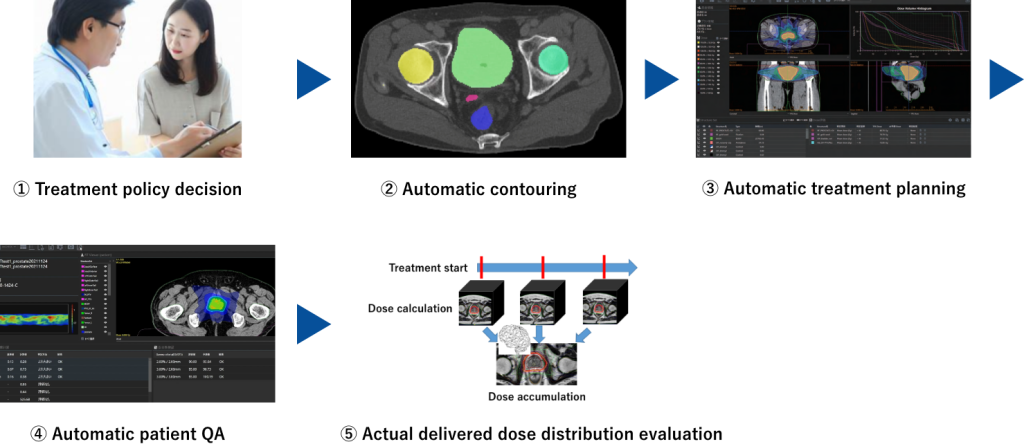
① Treatment policy decision
Using the latest image processing technology and large-scale patient cases (big data) that have been treated so far, we are conducting research to predict the prognosis (therapeutic effect) of radiation therapy with high accuracy, the occurrence of side effects, and the shrinkage of tumors before the start of treatment. This research field, also known as radiomics, is research that attempts to achieve unprecedentedly high-precision prognosis prediction from medical images alone. Domestic radiomics books written by many members of our laboratory have been published. So far, we have developed a new radiomics feature value, a technology to predict tumor shrinkage due to radiation therapy using deep learning, and a technology to predict radiation pneumonitis.
Key papers
・Tanaka S, Kadoya N, Sugai Y, Umeda M, Ishizawa M, Katsuta Y, Ito K, Takeda K, Jingu K, “A deep learning-based radiomics approach to predict head and neck tumor regression for adaptive radiotherapy”, Sci Rep. 2022 May 27;12(1):8899.
・Sugai Y, Kadoya N, Tanaka S, Tanabe S, Umeda M, Yamamoto T, Takeda K, Dobashi S, Ohashi H, Takeda K, Jingu K, “Impact of feature selection methods and subgroup factors on prognostic analysis with CT-based radiomics in non-small cell lung cancer patients”, Radiat Oncol. 2021 Apr 30;16(1):80.
・Kadoya N, Tanaka S, Kajikawa T, Tanabe S, Abe K, Nakajima Y, Yamamoto T, Takahashi N, Takeda K, Dobashi S, Takeda K, Nakane K, Jingu K, “Homology-based Radiomic Features for Prediction of the Prognosis of Lung Cancer Based on CT-based Radiomics”, Med Phys. 2020 Feb 25
・Tanaka S, Kadoya N, Kajikawa T, Matsuda S, Dobashi S, Takeda K, Jingu K. Investigation of thoracic four-dimensional CT-based dimension reduction technique for extracting the robust radiomic features. Phys Med. 2019 Feb;58:141-148.
Press release
Cup = ring? : New images created by homology analysis technology… | Press releases and research results | Tohoku University
Toward the Realization of Personalized Radiation Therapy Using Deep Learning to Treat Head and Neck Cancer | Press Releases and Research Results | Tohoku University
Radiomics is a coined word consisting of radio, which means radiation images, and omics, which indicates science that systematically handles large amounts of information. It is used to improve the quality of disease prevention, diagnosis, treatment and prognosis.
Grant
・The Terumo Life Science Foundation Research and Development Grant (April 2023-March 2024, research representative: Noriyuki Kadoya, 2 million yen)
・Grants-in-Aid for Scientific Research C “Research on clinical information and radiomics feature-guided adaptive radiation therapy for personalized medicine for head and neck cancer” (April 2021-March 2024, research representative: Ken Takeda, Research co-investigator: Noriyuki Kadoya) (Joint research with School of Health Sciences, Tohoku Univ)
・Grants-in-Aid for Scientific Research C “Development of a method to create lung function images from CT images only using radiomics technology” (April 2019-March 2023, research representative: Noriyuki Kadoya, 4.29 million yen)
・Grants-in-Aid for Scientific Research Support for starting research activities “Development of a method to predict tumor shrinkage from pre-treatment medical images for personalized medicine” (September 2020-March 2023, research representative: Shohei Tanaka, 2.73 million yen)
② Automatic contouring
We are researching high-precision automatic contouring technology using the latest image processing technology and large-scale cases (big data) of previously treated patients. Contouring is a necessary task for radiation therapy, and is the task of drawing the contours of tumors and organs at risk (OARs) in each slice of CT and MR images. It is not uncommon for a contour to take up to 3 hours per case for clinical sites with a lot of OARs. We aim to develop a technology that can automatically contour it using advanced image processing including AI, aiming to reduce operational efficiency and variations in contour creation among medical staff.
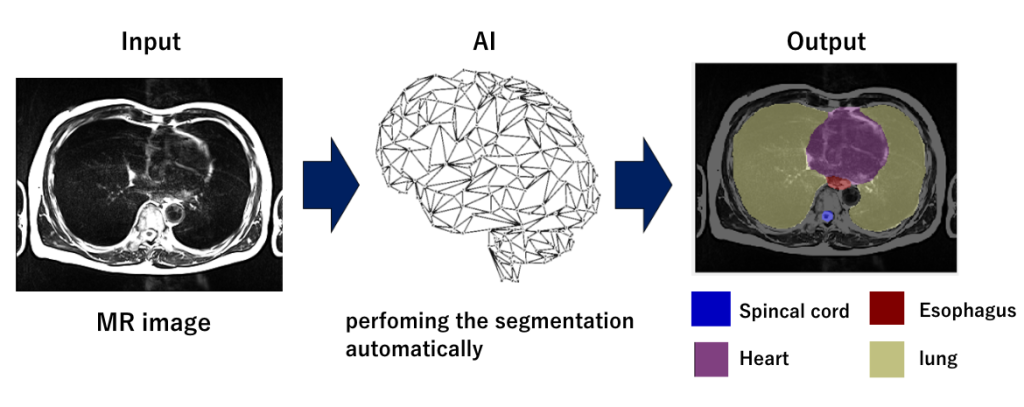
Grant
・ NEDO Entrepreneurs Program (NEP) Type B (August 2022 to March 2023, Airato Inc, 25 million yen, shared research)
・Iori Sumida et al. “Artificial Intelligence in Radiation Therapy (Ipem-iop Series in Physics and Engineering in Medicine and Biology”, Inst of Physics Pub Inc (2023/2/28) (Co-author, Noriyuki Kadoya)
Book
・Iori Sumida et al. “Artificial Intelligence in Radiation Therapy (Ipem-iop Series in Physics and Engineering in Medicine and Biology”, Inst of Physics Pub Inc (2023/2/28) (Co-author, Noriyuki Kadoya)
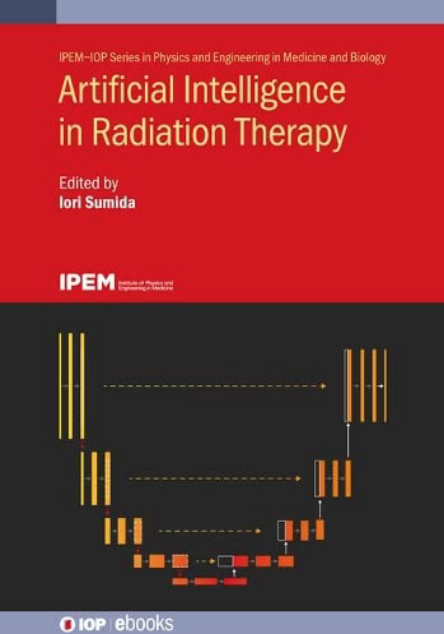
http://www.amazon.co.jp/Artificial-Intelligence-Radiation-Therapy-ebooks/dp/0750333375
③ Automatic treatment planning
We are developing technology that automatically generates optimal treatment plans using AI (deep learning). This technology generates an optimal treatment plan based on learned treatment plan data simply by inputting CT and contour information (figure below).
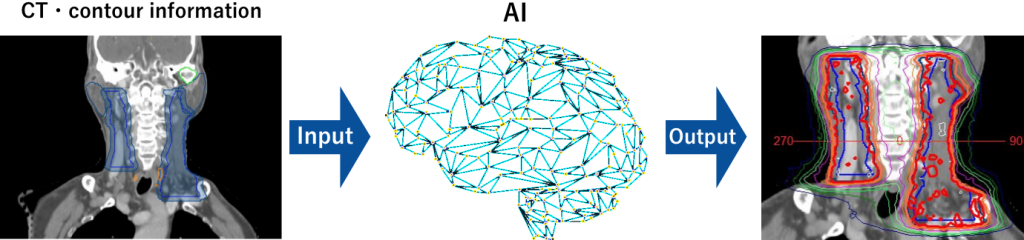
Regarding the technology to predict the optimal dose distribution, AI models have been developed for head and neck, chest, prostate, and cervical cancer, and are being implemented in society through industry-academia collaboration. In recent years, we have also begun developing next-generation AI models using deep reinforcement learning.
Key papers
・Kajikawa T, Kadoya N, Ito K, Takayama Y, Chiba T, Tomori S, Nemoto H, Dobashi S, Takeda K, Jingu K. A convolutional neural network approach for IMRT dose distribution prediction in prostate cancer patients. J Radiat Res. 2019 Oct 23;60(5):685-693. doi: 10.1093/jrr/rrz051. PMID: 31322704; PMCID: PMC6805973.
・Kajikawa T, Kadoya N, Ito K, Takayama Y, Chiba T, Tomori S, Takeda K, Jingu K. Automated prediction of dosimetric eligibility of patients with prostate cancer undergoing intensity-modulated radiation therapy using a convolutional neural network. Radiol Phys Technol. 2018 Sep;11(3):320-327.
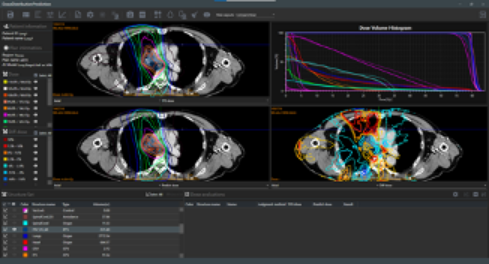
AI-based optimal dose distribution prediction system, AIVOT-QA (AiRato, Inc)
Product page (AiRato, Inc)
Media
・ TV Tokyo World Business Satellite (WBS) “Cancer treatment evolving with AI Latest technology x Future of medical care https://www.tv-tokyo.co.jp/mv/wbs/feature/post_273425/ (2023.5.4)
Grant
・The Terumo Life Science Foundation Research and Development Grant (April 2023-March 2024, research representative: Noriyuki Kadoya, 2 million yen)
Grants-in-Aid for Scientific Research Fundamental C “Development of true ‘artificial intelligence-type’ automatic irradiation planning method by deep reinforcement learning” (April 2023-March 2026, research representative: Noriyuki Kadoya, 5 million yen)
・ NEDO Entrepreneurs Program (NEP) Type B (August 2022 to March 2023, Airato Inc, 25 million yen, shared research)
④ Automatic patient QA
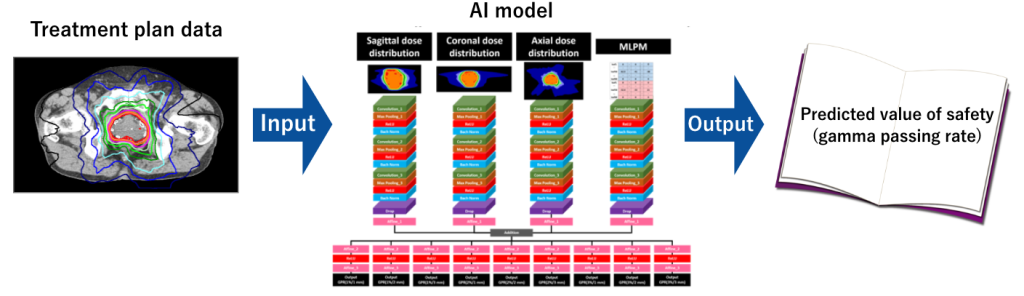
AI models have already been developed for the head and neck and prostate, and are being implemented in society through industry-academia collaboration. In recent years, we have been developing new optimal input values that accurately predict safety verification results from treatment plan data.
Key papers
・Tozuka
・Tomori S, Kadoya N, Kajikawa T, Kimura Y, Narazaki K, Ochi T, Jingu K. Systematic method for a deep learning-based prediction model for gamma evaluation in patient-specific quality assurance of volumetric modulated arc therapy. Med Phys. 2021 Mar;48(3):1003-1018. doi: 10.1002/mp.14682. Epub 2021 Jan 28. PMID: 33368406.
・Kimura Y, Kadoya N, Tomori S, Oku Y, Jingu K. Error detection using a convolutional neural network with dose difference maps in patient-specific quality assurance for volumetric modulated arc therapy. Phys Med. 2020 May;73:57-64.
・Tomori S, Kadoya N, Takayama Y, Kajikawa T, Shima K, Narazaki K, Jingu K. A deep learning-based prediction model for gamma evaluation in patient-specific quality assurance. Med Phys. 2018 Jul 31.
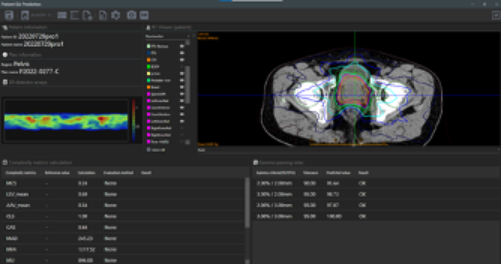
AI-based automatic patient QA system, AIVOT-QA (AiRato, Inc)
Product page (AiRato, Inc)
Grant
・ NEDO Entrepreneurs Program (NEP) Type B (August 2022 to March 2023, Airato Inc, 25 million yen, shared research)
⑤ Actual delivered dose distribution evaluation
Using AI (deep learning) and log files, we are researching a real dose distribution evaluation method for patients that reflects changes in internal organs and mechanical movements during irradiation (figure below). This makes it possible to evaluate the real delivered dose to patients in on-line ART, which can be performed with MR-Linac.
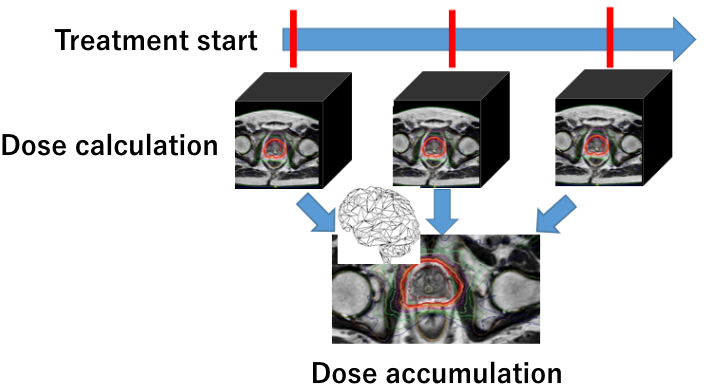
Key papers
・Katsuta Y, Kadoya N, Fujita Y, Shimizu E, Majima K, Matsushita H, Takeda K, Jingu K. Log file-based patient dose calculations of double-arc VMAT for head-and-neck radiotherapy. Phys Med. 2018 Apr;48:6-10. doi: 10.1016/j.ejmp.2018.03.006. Epub 2018 Mar 20. PMID: 29728230.
・Kadoya N, Kon Y, Takayama Y, Matsumoto T, Hayashi N, Katsuta Y, Ito K, Chiba T, Dobashi S, Takeda K, Jingu K. Quantifying the performance of two different types of commercial software programs for 3D patient dose reconstruction for prostate cancer patients: Machine log files vs. machine log files with EPID images. Phys Med. 2018 Jan;45:170-176.
・Katsuta Y, Kadoya N, Fujita Y, Shimizu E, Matsunaga K, Matsushita H, Majima K, Jingu K. Clinical impact of dosimetric changes for volumetric modulated arc therapy in log file-based patient dose calculations. Phys Med. 2017 Oct;42:1-6.
・Katsuta Y, Kadoya N, Fujita Y, Shimizu E, Matsunaga K, Matsushita H, Majima K, Jingu K. Quantification of residual dose estimation error on log file-based patient dose calculation. Phys Med. 2016 May;32(5):701-5.
Grant
・Grants-in-Aid for Scientific Research Fundamental C “Creation of real dose distribution considering movement during irradiation by deep learning technology” (April 2023-March 2028, research representative: Shohei Tanaka, 4.81 million yen)
2. Development of new image using AI/DIR
① CT-based lung functional imaging
It has been reported that it is possible to reduce lung damage and effectively irradiate tumors by applying lung function imaging to make the treatment plan for avoiding areas with high lung function. In addition to the method using four-dimensional tomography (4D-CT) images proposed by us, there are conventional methods for acquiring pulmonary function images include nuclear medicine images, enzymatic gas-enhanced MRI, and CT images using xenon gas. However, these methods are not widely used due to the reasons such as long time, low resolution, and high cost. Therefore, the method we propose is a novel method that uses 4D-CT and deformable image registration (DIR) image processing technology to create lung function images based on lung volume changes. It is a method to acquire functional images, and it is thought that this method can solve the problems of acquiring lung function images described above (see figure below). First, by using only 4D-CT images aquired by CT scanner installed in many hospitals, there is no need for additional examinations, and high-resolution functional images can be quickly created from “free” information. Due to this advantage, it is likely to be more widely accepted than the currently used method of acquiring pulmonary function images, and it is expected that many facilities will be able to realize pulmonary function-based radiation therapy. Our research group examines the effectiveness of radiation therapy using pulmonary function images created from 4D-CT and DIR, evaluates the accuracy of DIR, evaluates the accuracy of lung function, and develops and analyzes for clinical use. In recent years, we have started clinical trials using this pulmonary function image in joint research with Hiroshima University and Kochi University. Assuming clinical use, we are also developing a variable lung phantom for DIR verification.
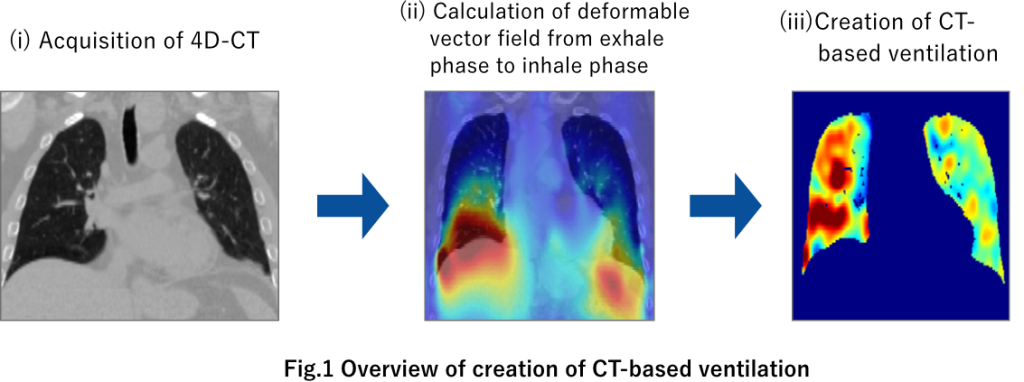
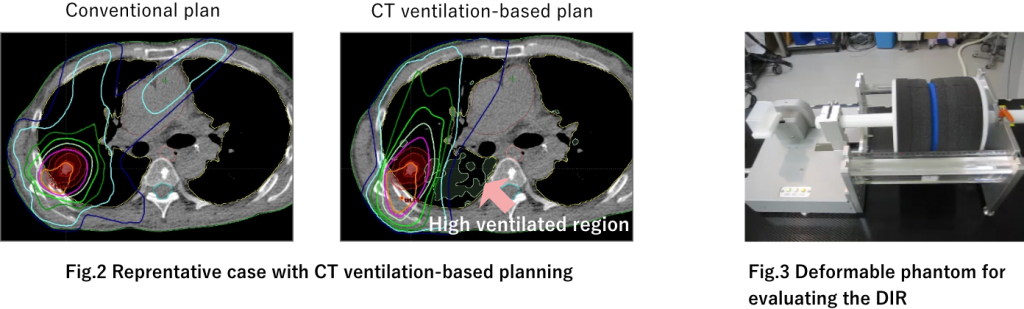
・Ieko Y, Kadoya N, Sugai Y, Mouri S, Umeda M, Tanaka S, Kanai T, Ichiji K, Yamamoto T, Ariga H, Jingu K. Assessment of a computed tomography-based radiomics approach for assessing lung function in lung cancer patients. Phys Med. 2022 Sep;101:28-35.
・ Kajikawa T, Kadoya N, Maehara Y, Miura H, Katsuta Y, Nagasawa S, Suzuki G, Yamazaki H, Tamaki N, Yamada K. A deep learning method for translating 3DCT to SPECT ventilation imaging: First comparison with 81m Kr-gas SPECT ventilation imaging. Med Phys. 2022 Jul;49(7):4353-4364
・Kadoya N, Nemoto H, Kajikawa T, Nakajima Y, Kanai T, Ieko Y, Ikeda R, Sato K, Dobashi S, Takeda K, Jingu K. Evaluation of four-dimensional cone beam computed tomography ventilation images acquired with two different linear accelerators at various gantry speeds using a deformable lung phantom. Phys Med. 2020 Sep;77:75-83.
・Kipritidis J, Tahir BA, Cazoulat G, Hofman MS, Siva S, Callahan J, Hardcastle N, Yamamoto T, Christensen GE, Reinhardt JM, Kadoya N, Patton TJ, Gerard SE, Duarte I, Archibald-Heeren B, Byrne M, Sims R, Ramsay S, Booth JT, Eslick E, Hegi-Johnson F, Woodruff HC, Ireland RH, Wild JM, Cai J, Bayouth JE, Brock K, Keall PJ. The VAMPIRE challenge: A multi-institutional validation study of CT ventilation imaging. Med Phys. 2019 Mar;46(3):1198-1217.
・Faught AM, Miyasaka Y, Kadoya N, Castillo R, Castillo E, Vinogradskiy Y, Yamamoto T. Evaluating the Toxicity Reduction With Computed Tomographic Ventilation Functional Avoidance Radiation Therapy. Int J Radiat Oncol Biol Phys. 2017 Oct 1;99(2):325-333.
・Kanai T, Kadoya N, Ito K, Kishi K, Dobashi S, Yamamoto T, Umezawa R, Matsushita H, Takeda K, Jingu K. Evaluation of four-dimensional computed tomography (4D-CT)-based pulmonary ventilation: The high correlation between 4D-CT ventilation and (81m)Kr-planar images was found. Radiother Oncol. 2016 Jun;119(3):444-8.
Grant
・Grant-in-Aid for Scientific Research B “Development of custom-made radiation therapy using lung function imaging for locally advanced non-small cell lung cancer” (April 2020-March 2024, research representative Tomoki Kimura, research co-investigator: Noriyuki Kadoya) (Joint research with Hiroshima University and Kochi University)
・Grants-in-Aid for Scientific Research C “Clinical application of custom-made radiation therapy using functional imaging for lung cancer” (April 2017-March 2020, research representative: Tomoki Kimura, research co-investigator: Noriyuki Kadoya)
・Tohoku University Hospital Clinical Application Research Promotion Program by Young Researchers (April 2015-March 2017, Research Representative: Noriyuki Kadoya, 2 million yen)
・Japan Radiological Society Bayer Research Grant (April 2014-March 2015, research representative: Noriyuki Kadoya, 1 million yen)
・Grants-in-Aid for Young Scientists B “Development of pulmonary function images using four-dimensional tomography and application to radiotherapy” (April 2012-March 2014, research representative: Noriyuki Kadoya, 3.9 million yen)
② Radiation pneumonitis hazard map
Using CT images and AI, we are developing a “hazard map” that predicts the risk of serious radiation pneumonitis due to radiation exposure (figure below). By applying this technology to VMAT (Volumetric Modulated Arc Therapy), it is possible to reduce the radiation exposure of lung tissue indicated as dangerous on the hazard map. This radiotherapy is expected to make lung cancer radiotherapy less invasive and improve its therapeutic effect.
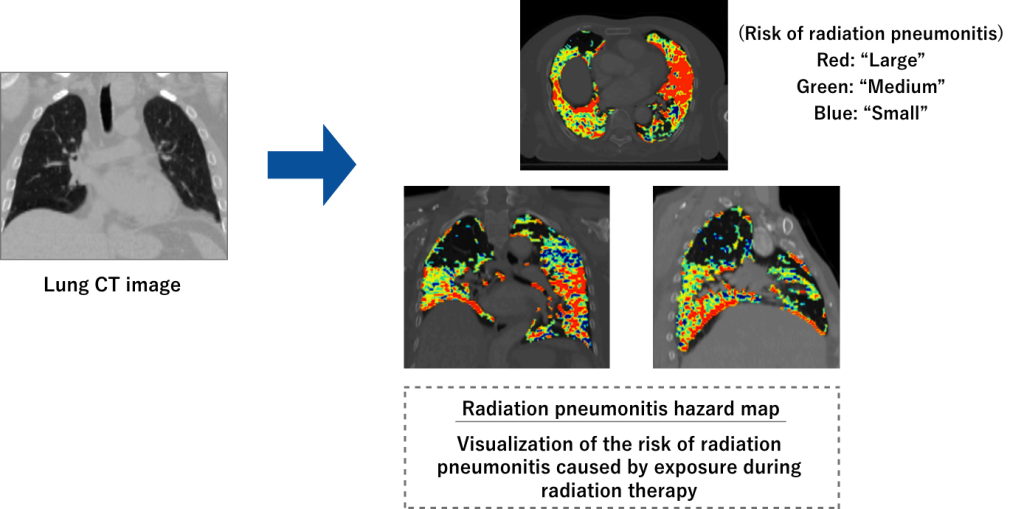
・Katsuta Y, Kadoya N, Kajikawa T, Mouri S, Kimura T, Takeda K, Yamamoto T, Imano N, Tanaka S, Ito K, Kanai T, Nakajima Y, Jingu K. Radiation pneumonitis prediction model with integrating multiple dose-function features on 4DCT ventilation images. Phys Med. 2023 Jan;105:102505.
・Katsuta Y, Kadoya N, Sugai Y, Katagiri Y, Yamamoto T, Takeda K, Tanaka S, Jingu K. Feasibility of Differential Dose-Volume Histogram Features in Multivariate Prediction Model for Radiation Pneumonitis Occurrence. Diagnostics (Basel). 2022 May 31;12(6):1354.
・Katsuta Y, Kadoya N, Mouri S, Tanaka S, Kanai T, Takeda K, Yamamoto T, Ito K, Kajikawa T, Nakajima Y, Jingu K. Prediction of radiation pneumonitis with machine learning using 4D-CT based dose-function features. J Radiat Res. 2022 Jan;63:71-79.
Grant
・Grants-in-Aid for Young Scientists B “Construction of radiation pneumonitis prediction model that integrates dose evaluation of lung function and morphology” (April 2020-March 2024, research representative Yoshiyuki Katsuta, 2.99 million yen)
3. Clinical evaluation and technical development of automatic contouring and accumulated dose distribution evaluation system using deformable rigid registration
We are conducting clinical evaluation and technical development of automatic contouring and accumulated dose distribution evaluation system using deformable rigid registration (DIR). By utilizing DIR between CT-CT, CT-MR, and MR-MR, contours can be automatically generated on CT and MR images, and the dose distribution calculated for each image can be added with high accuracy. This technology allows for faster contouring and more accurate patient dose assessment. We are actively conducting clinical use and research at RALS and MR-Linac (figure below). DIR books in the field of radiotherapy, which are written by many members of our laboratory, have also been published.
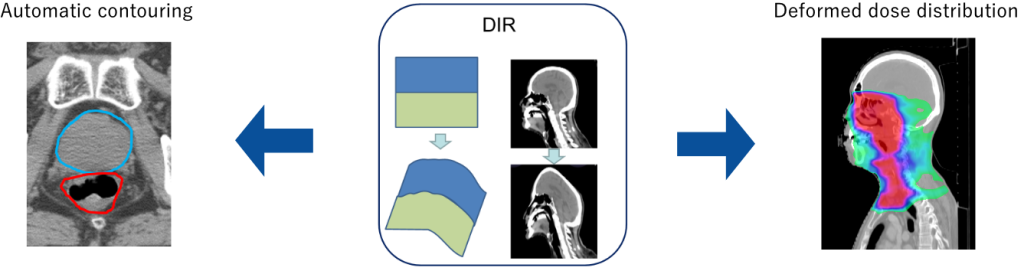
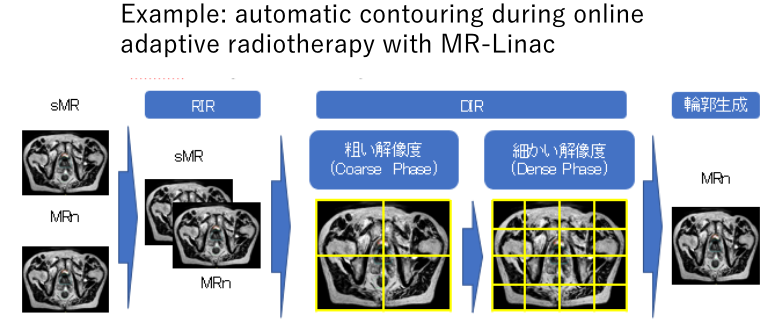
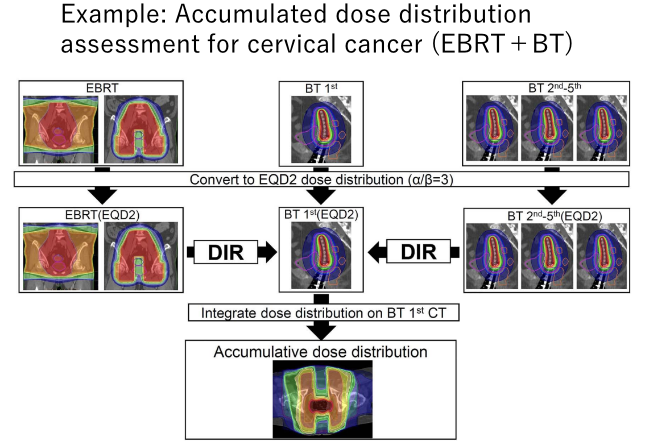
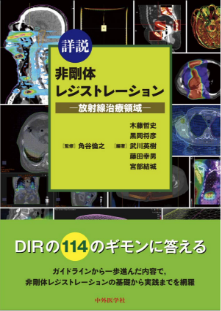
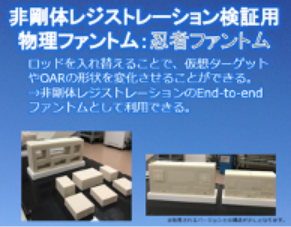
・Miyasaka Y, Kadoya N, Umezawa R, Takayama Y, Ito K, Yamamoto T, Tanaka S, Dobashi S, Takeda K, Nemoto K, Iwai T, Jingu K. Comparison of predictive performance for toxicity by accumulative dose of DVH parameter addition and DIR addition for cervical cancer patients. J Radiat Res. 2021 Jan 1;62(1):155-162.
・Sugawara Y, Kadoya N, Kotabe K, Nakajima Y, Ikeda R, Tanabe S, Ohashi H, Jingu K. Development of a dynamic deformable thorax phantom for the quality management of deformable image registration. Phys Med. 2020 Sep;77:100-107.
・Kadoya N, Miyasaka Y, Nakajima Y, Kuroda Y, Ito K, Chiba M, Sato K, Dobashi S, Yamamoto T, Takahashi N, Kubozono M, Takeda K, Jingu K. Evaluation of deformable image registration between external beam radiotherapy and HDR brachytherapy for cervical cancer with a 3D-printed deformable pelvis phantom. Med Phys. 2017 Apr;44(4):1445-1455.
・Kadoya N, Nakajima Y, Saito M, Miyabe Y, Kurooka M, Kito S, Fujita Y, Sasaki M, Arai K, Tani K, Yagi M, Wakita A, Tohyama N, Jingu K. Multi-institutional Validation Study of Commercially Available Deformable Image Registration Software for Thoracic Images. Int J Radiat Oncol Biol Phys. 2016 Oct 1;96(2):422-431.
・Kadoya N, Fujita Y, Katsuta Y, Dobashi S, Takeda K, Kishi K, Kubozono M, Umezawa R, Sugawara T, Matsushita H, Jingu K. Evaluation of various deformable image registration algorithms for thoracic images. J Radiat Res. 2014 Jan 1;55(1):175-82.
Grant
・Grants-in-Aid for Scientific Research Fostering International Collaborative Research B “Creation and dissemination of advanced technology in radiotherapy centered on the international medical physics network” (October 2018-March 2023, principal investigator Mitsuhiro Nakamura, co-investigator: Noriyukii Kadoya) (Collaboration with Nakamura Laboratory, Graduate School of Medicine, Kyoto University)
・Grants-in-Aid for Young Scientists B “Development of highly accurate integrated dose evaluation system for external irradiation and intracavitary irradiation for cervical cancer” (April 2015-March 2019, research representative Noriyuki Kadoya, 4.03 million yen)
(Joint research with Oshiro Lab., Osaka University)
・Microselectron HDR research group research grant (January 2014-December 2015, research representative: Noriyuki Kadoya, 1 million yen)
4. Clinical technology research of online adaptive radiotherapy with MR-Linac
We are investigating optimal treatment planning methods using MR-Linac, developing optimal dose calculation methods using MR images, and developing end-to-end phantoms for online adaptive radiotherapy (see figure below).
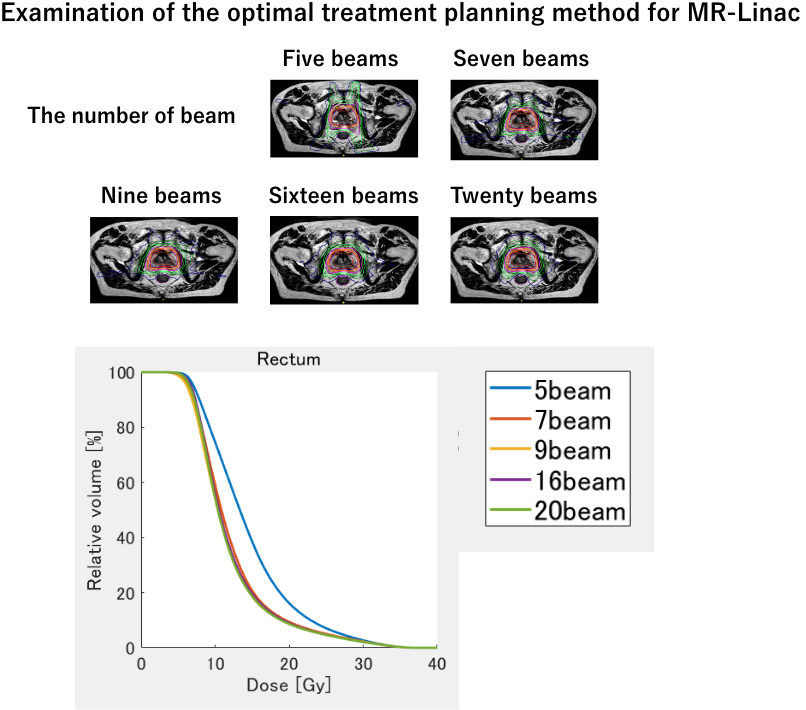
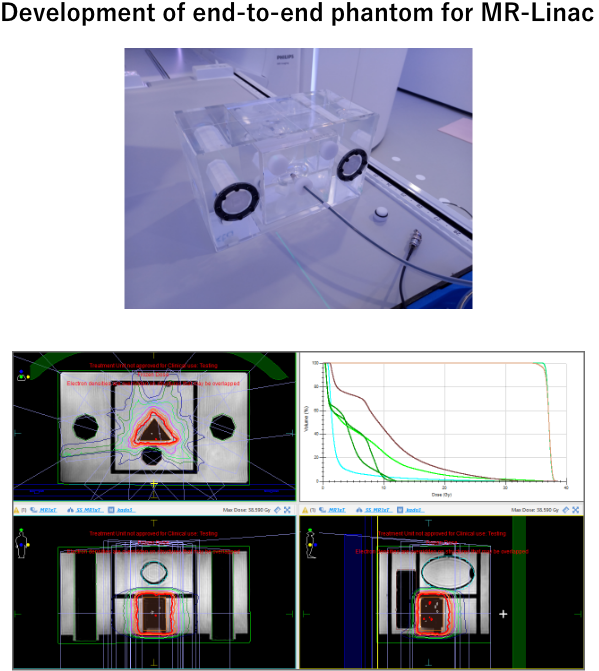
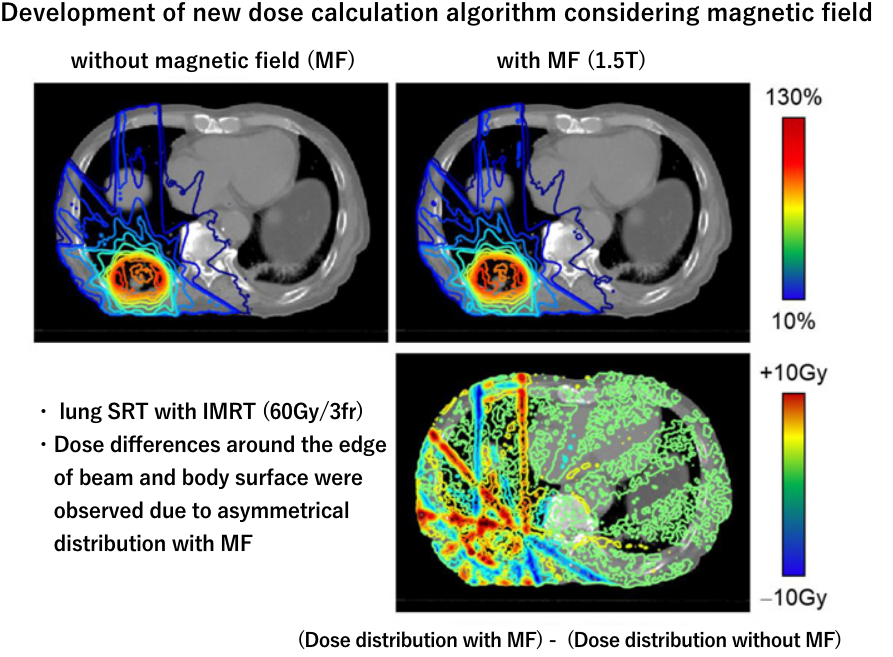
・Okamoto H, Igaki H, Chiba T, Shibuya K, Sakasai T, Jingu K, Inaba K, Kuroda K, Aoki S, Tatsumi D, Nakamura M, Kadoya N, Furuyama Y, Kumazaki Y, Tohyama N, Tsuneda M, Nishioka S, Itami J, Onishi H, Shigematsu N, Uno T. Practical guidelines of online MR-guided adaptive radiotherapy. J Radiat Res. 2022 Sep 21;63(5):730-740.
・Ito K, Kadoya N, Katsuta Y, Tanaka S, Dobashi S, Takeda K, Jingu K. Evaluation of the electron transport algorithm in magnetic field in EGS5 Monte Carlo code. Phys Med. 2022 Jan;93:46-51.
・Kajikawa T, Kadoya N, Tanaka S, Nemoto H, Takahashi N, Chiba T, Ito K, Katsuta Y, Dobashi S, Takeda K, Yamada K, Jingu K. Dose distribution correction for the influence of magnetic field using a deep convolutional neural network for online MR-guided adaptive radiotherapy. Phys Med. 2020 Dec;80:186-192.
Grant
・Grant-in-Aid for Young Researcher “Development of Nonlinear CCC Dose Calculation Algorithm for MRI-Linac” (April 2018-March 2020, Research Representative Kengo Ito, 3.51 million yen)
・ Grants-in-Aid for Scientific Research Young (B) “Development of a high-precision dose calculation system using quantitative magnetic susceptibility mapping” (April 2015-March 2017, research representative Kengo Ito, 4.03 million yen)